Swin Transformer: Hierarchical Vision Transformer using Shifted Windows
主要贡献:
Patch Merging Layer

Shifted Window
标准Transformer是计算全局注意力图,即计算每个token和所有token的相关性,因此复杂度是token数量的二次方;本文提出在局部区域计算注意力图,将全局分为M✖️M个无重叠局部,将复杂度降低。
Method | Image size | Param (M) | GFLOPs | Top1 err |
ResNet50 | 2242 | 25.6 | 4.1 | 21.5 |
ResNeXt50 | 2242 | 25.0 | 4.3 | 20.5 |
DeiT-S | 2242 | 22.1 | 4.6 | 20.2 |
PVT-S | 2242 | 24.5 | 3.8 | 20.2 |
Swin-T | 2242 | 29 | 4.5 | 18.7 |
Method | Image size | Param (M) | GFLOPs | Top1 Err |
ResNet101 | 2242 | 44.7 | 7.9 | 20.2 |
ResNeXt101 | 2242 | 44.2 | 8.0 | 19.4 |
ViT-S | 2242 | 48.8 | 9.9 | 19.2 |
PVT-M | 2242 | 44.2 | 6.7 | 18.8 |
Swin-S | 2242 | 50 | 8.7 | 17.0 |
Method | Image size | Param (M) | GFLOPs | Top1 err |
ResNeXt101 | 2242 | 83.5 | 15.6 | 18.5 |
ViT-B | 2242 | 86.6 | 17.6 | 18.2 |
DeiT-B | 2242 | 86.6 | 17.6 | 18.2 |
PVT-L | 2242 | 61.4 | 9.8 | 18.3 |
Swin-B | 2242 | 88 | 15.4 | 16.7 |
Swin-B | 3842 | 88 | 47 | 15.8 |
Swin-B(22k) | 3842 | 88 | 47 | 14 |
Swin-L(22k) | 3842 | 197 | 104 | 13.6 |
局部注意力图缺失了全局信息交换能力,因此需要加入跨区块连接。如图所示,第一层使用从左上角开始分块的标准方式,第二层将分块起点偏移「M/2, M/2」。
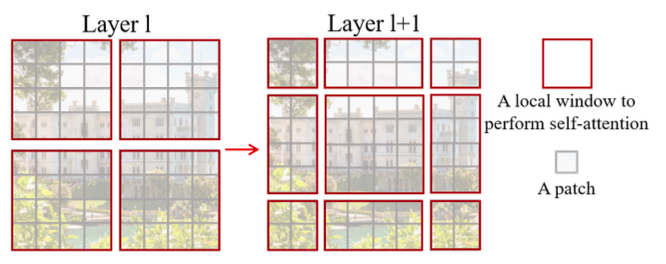
分块起点的偏移会导致分块数量增加、分块的大小不同,本文使用循环偏移方式。如图在偏移后,将边缘的不相邻的子窗口拼接,保持分块大小不变、数量不变;然后在计算时使用mask机制限制self-attention在每个子区域中。
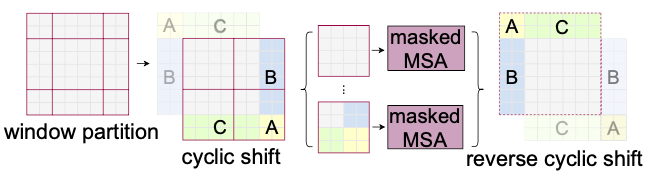
# cyclic shift
shifted_x = torch.roll(x, shifts=(-self.shift_size, -self.shift_size), dims=(1, 2))
# W-MSA/SW-MSA
attn_windows = self.attn(x_windows, mask=self.attn_mask)
# cycle shift back
x = torch.roll(shifted_x, shifts=(self.shift_size, self.shift_size), dims=(1, 2))
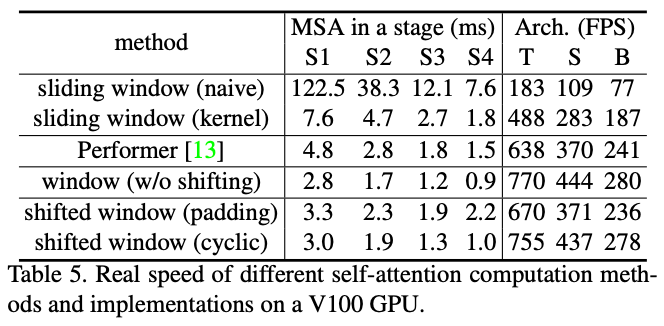
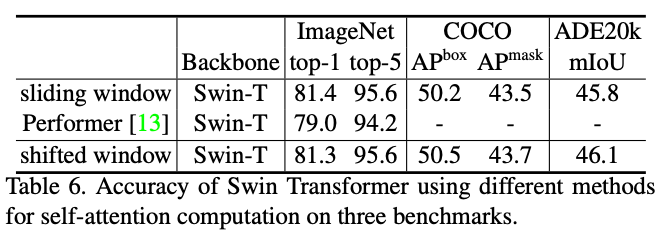
在self-attention结构上,与Performer相比更快更准,与sliding window相比更快、同时精度相似。
Relative Position Bias
本文在计算self-attention时,给相关性的头部增加了相对位置偏置B,即:
Attention(Q,K,V)=SoftMax(QK^T/\sqrt d +B)V, B \in \R^{M^2\times M^2}
# 每个局部Attention增加一个自学习的relative_position_bias_table
# 尺寸为(7x7, nH),定义如下:
relative_position_bias_table = nn.Parameter(
torch.zeros((2 * window_size[0] - 1) * (2 * window_size[1] - 1), num_heads))
trunc_normal_(relative_position_bias_table, std=.02)
# 对每个token建立局部qk的距离索引表
# 尺寸为(7x7, 7x7),定义如下:
coords_h = torch.arange(self.window_size[0])
coords_w = torch.arange(self.window_size[1])
coords = torch.stack(torch.meshgrid([coords_h, coords_w])) # 2, Wh, Ww
coords_flatten = torch.flatten(coords, 1) # 2, Wh*Ww
relative_coords = coords_flatten[:, :, None] - coords_flatten[:, None, :] # 2, Wh*Ww, Wh*Ww
relative_coords = relative_coords.permute(1, 2, 0).contiguous() # Wh*Ww, Wh*Ww, 2
relative_coords[:, :, 0] += self.window_size[0] - 1 # shift to start from 0
relative_coords[:, :, 1] += self.window_size[1] - 1
relative_coords[:, :, 0] *= 2 * self.window_size[1] - 1
relative_position_index = relative_coords.sum(-1) # Wh*Ww, Wh*Ww
self.register_buffer("relative_position_index", relative_position_index)
# 查询table得到bias值
relative_position_bias = self.relative_position_bias_table[self.relative_position_index.view(-1)].view(
self.window_size[0] * self.window_size[1], self.window_size[0] * self.window_size[1], -1) # Wh*Ww,Wh*Ww,nH
relative_position_bias = relative_position_bias.permute(2, 0, 1).contiguous() # nH, Wh*Ww, Wh*Ww
相关资料:
预训练模型中的可学习相对位置偏置B,可以通过bi-cubic插值,用于初始化不同窗口尺寸的模型微调。
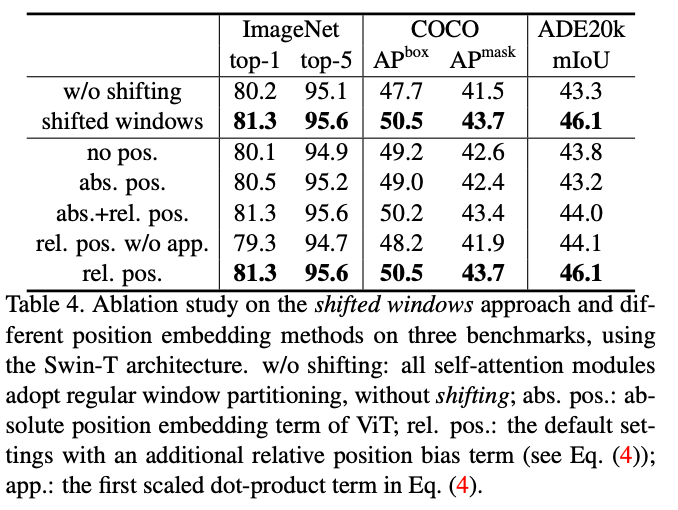
实验结果
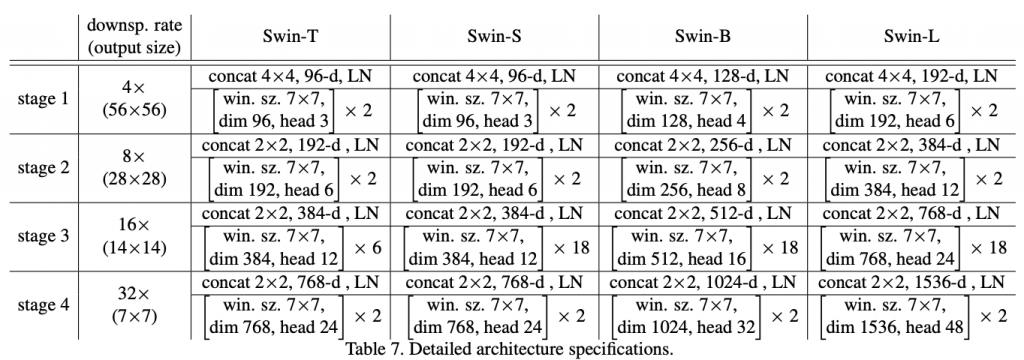
仍然尺度敏感
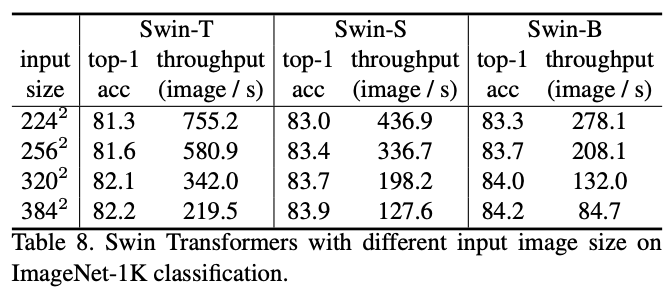
ImageNet分类
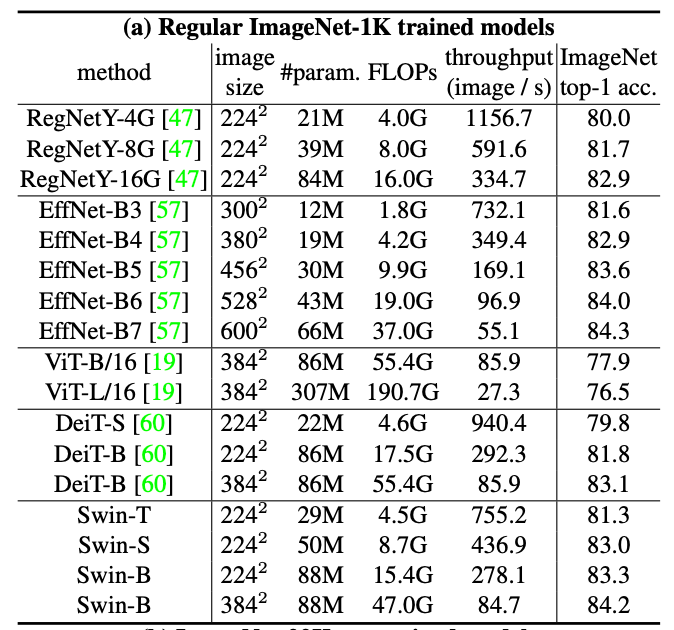
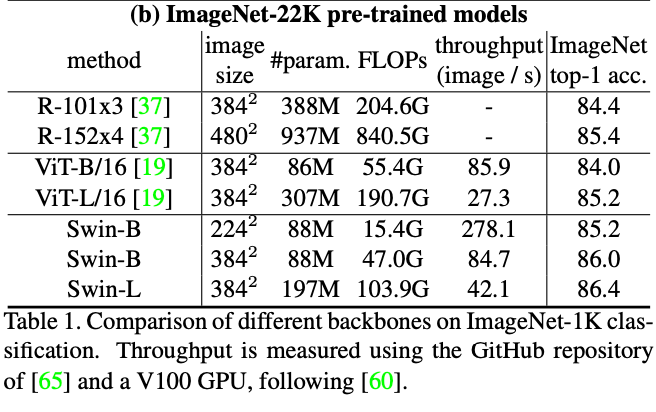
MSCOCO目标检测结果(SOTA+2.7boxAP/2.3msakAP)
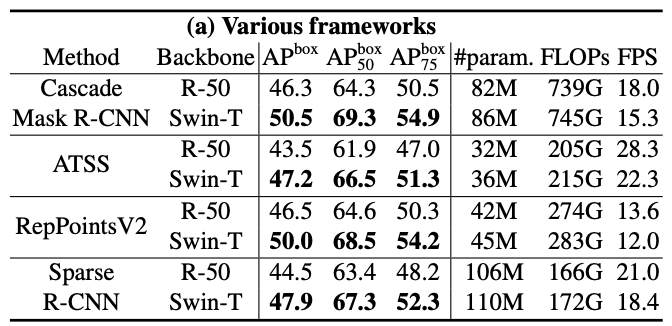
ResNet-50 vs Swin-Transformer-T
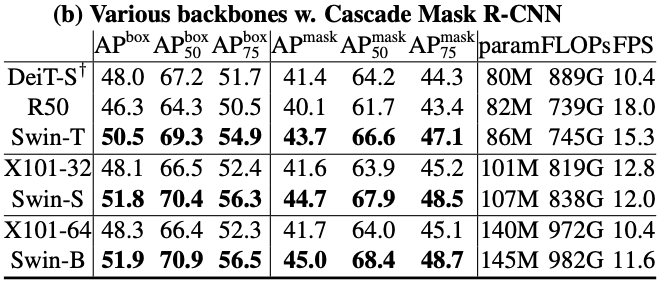
- transformer networks:DeiT
- standard ConvNets:ResNeXt
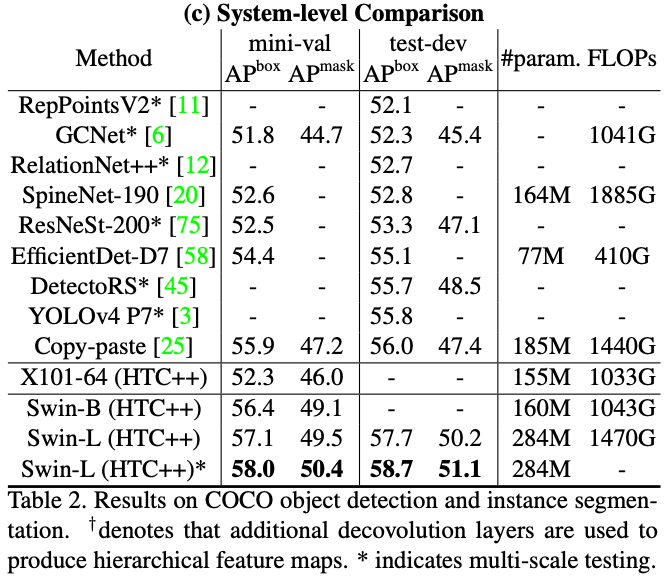
ADE20K语义分割结果
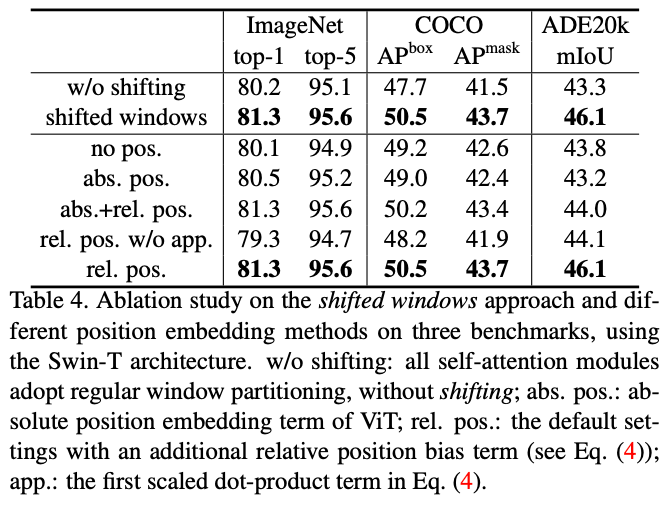
其他Tips
- 从语言领域到视觉领域,主要的不同在于
- 尺度差异大,目前tokens都是固定尺度,可能不适用于视觉任务
- 像素分辨率更高,因此计算复杂度与图像尺寸成O2
- DeiT介绍了多种训练策略,使ViT在小数据集上也能更有效
- PVT的计算复杂度与图像尺寸仍是O2
- 对于ResNeXt网络,使用AdamW相比SGD精度更高
- repeated augmentation对于ViT的稳定训练不重要
6666